Microsoft Power BI is not just a single method. It’s important to consider this as you prepare your approach to use this software. Power BI is a series of resources, instead. These resources have started their lives as distinct organizations that reside in a few different environments. After multiple iterations and machinations, Microsoft has put these tools together in a single environment—the tool chest that we call Power BI.
Power BI is one of the unusual instances where the pieces work exceptionally well together. The business intelligence (BI) infrastructure process flows well from one tool to the next, and back as needed.
The BI Process
Before looking directly at the tools in the Power BI tool chest, we need to take a quick look at the basic BI method. How do we take data that is developed and handled by our line of business systems—our enterprise resource planning (ERP) systems, our accounting systems, our human resource systems, and so on—and place it in an environment where we can use it to gain insights into our entire organization? There are five key steps to this method, as shown in Figure below
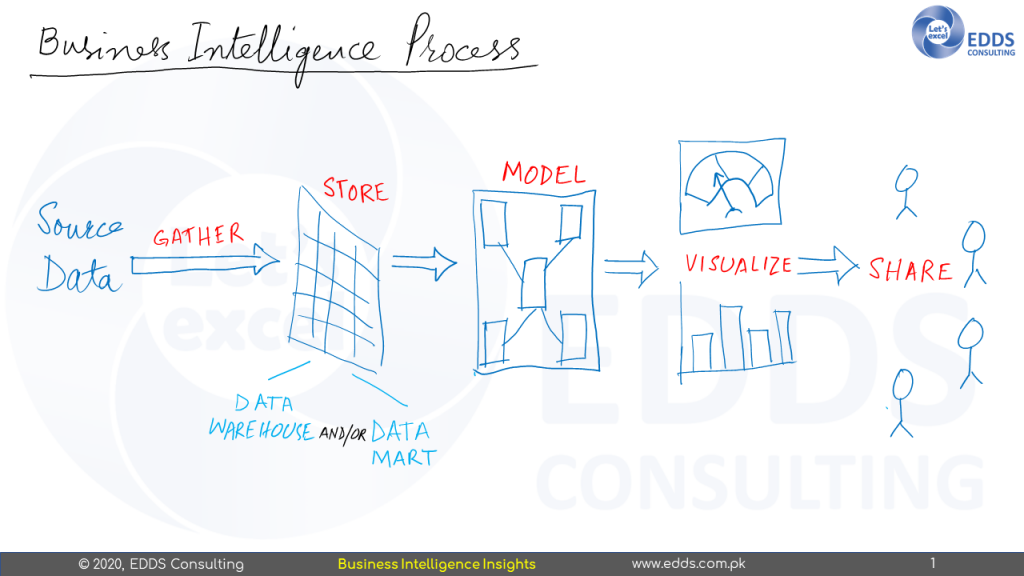
Gather
For ease and speed of study, data is collected from different sources and placed in a single location. When data is processed, it is also transformed. This transformation results in data that is free from errors and inconsistencies. The transformation also aligns the data with the rest of the data store, allowing it to function as a cohesive whole.
Store
Transformed data is stored at a particular location. This data storage is also referred to as a data store or data mart. The distinction between the two is just a matter of distance. The data warehouse aims to collect data from all over the organisation, whilst the data mart collects data that is confined to a specific subject within the organisation, such as sales or production.
Data stored in a data center or data mart is stored in a customized format specifically designed to allow for rapid retrieval during reporting and analysis. It is also organized in such a way that thousands or millions of individual transactions can be effectively combined (aggregated) to calculate, for example, the number of goods produced in a given month or the overall revenue in a given year. This type of data store is said to be designed for on-line analytical processing (OLAP).
Model
The data model is designed to serve two purposes on the top of the data store. First, it introduces the data to the business user in a way they’re familiar with—no odd table and field names to decipher, and no complicated language needed to query the data.
Second, it offers a single location for the storage of business calculations. The internal formulas used to calculate such items as the cost of products sold, the mean time between failure and days outside the time limit, can be put in a single location. When these calculations are required inside a report or on a dashboard, they are taken from a data model, not re-created over and over again.
Visualize
Once the data is usable in the model, it will be ready for review and exploration. This is achieved by developing maps, graphs, and gages for reports and dashboards. Some data visualizations may be generated for one-time use as a data model is being explored to address a very specific query. Other visualizations would be generated so that they can be shared with multiple users and referred to again and again.
Share
For those visualizations that are intended to be shared, we need a framework for distribution around the enterprise. A shared place is created where users can go and find the reports they need. This location has the necessary protection to ensure that users can only see the data for which they are allowed.
No Comments Yet